Ziyang Wang
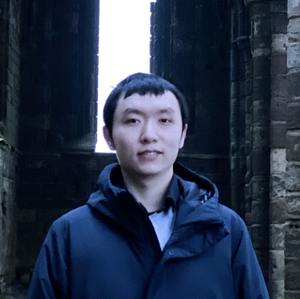
Biography
I completed my DPhil degree in Computer Science at the University of Oxford in 2024, MRes (Master by Research) degree with distinction at Imperial College London in 2018, and BEng degree from Xi’an Jiaotong University in 2017.
I keep my personal website up to date: [Personal Website], [GitHub], [ORCID].