Atılım Güneş Baydin
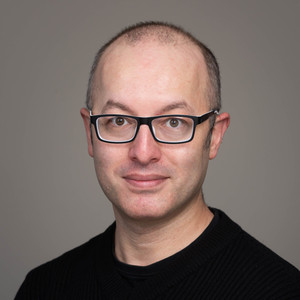
Dr Atılım Güneş Baydin
Wolfson Building, Parks Road, Oxford OX1 3QD
Interests
For up-to-date information please visit my personal page.
Differentiable programming, probabilistic programming, simulation-based inference, Bayesian methods
Biography
I am a Departmental Lecturer in Machine Learning at the Department of Computer Science and a Lecturer in Computer Science at Jesus College, University of Oxford, where I lead the Oxford AI4Science Lab.
I am also a member of the Torr Vision Group (TVG) at the Department of Engineering Science and the Oxford Applied and Theoretical Machine Learning Group (OATML). I am a Research Member of the Common Room at Kellogg College, a research consultant for Microsoft Research Cambridge, and a member of the European Lab for Learning and Intelligent Systems (ELLIS) Oxford Unit.
My work is at the intersection of generative modeling, probabilistic programming, and deep learning, with an interest in applications of machine learning for scientific discovery. I have recently focused on Bayesian inference in large-scale simulators, implementing code for distributed training and inference at supercomputing scale in collaboration with Lawrence Berkeley Lab. I am also involved in NASA and ESA Frontier Development Lab programs as faculty and member of the AI Technical Committee.
Previously I was a postdoc at Oxford working with Frank Wood on probabilistic programming. Before my work in Oxford, I was a postdoc with Barak Pearlmutter at the Brain and Computation Lab, National University of Ireland Maynooth. In Ireland I specialized in differentiable programming (automatic differentiation or “autodiff”) and I worked on compositionality, higher-order functions, and nesting of forward and reverse differentiation.
I have a PhD in artificial intelligence from Universitat Autònoma de Barcelona, where I was supervised by Ramon Lopez de Mantaras at the Artificial Intelligence Research Institute (IIIA) of the Spanish National Research Council (CSIC), working on computational analogy, commonsense reasoning, and graph-based evolutionary algorithms. I received my bachelor’s degree from Middle East Technical University and a master’s degree from Chalmers University of Technology, where I was working on artificial life and computational physics in the Complex Adaptive Systems program.
Selected Publications
-
Toward 3D Retrieval of Exoplanet Atmospheres: Assessing Thermochemical Equilibrium Estimation Methods
Michael D. Himes‚ Josepth Harrington and Atılım Güneş Baydin
In The Planetary Science Journal. Vol. 4. No. 74. 2023.
Details about Toward 3D Retrieval of Exoplanet Atmospheres: Assessing Thermochemical Equilibrium Estimation Methods | BibTeX data for Toward 3D Retrieval of Exoplanet Atmospheres: Assessing Thermochemical Equilibrium Estimation Methods | DOI (10.3847/PSJ/acc939) | Link to Toward 3D Retrieval of Exoplanet Atmospheres: Assessing Thermochemical Equilibrium Estimation Methods
-
Toward the End−to−End Optimization of Particle Physics Instruments with Differentiable Programming
Tommaso Dorigo‚ Andrea Giammanco‚ Pietro Vischia‚ Max Aehle‚ Mateusz Bawaj‚ Alexey Boldyrev‚ Pablo de Castro Manzano‚ Denis Derkach‚ Julien Donini‚ Auralee Edelen‚ Federica Fanzago‚ Nicolas R. Gauger‚ Christian Glaser‚ Atılım Güneş Baydin‚ Lukas Heinrich‚ Ralf Keidel‚ Jan Kieseler‚ Claudius Krause‚ Maxime Lagrange‚ Max Lamparth‚ Lukas Layer‚ Gernot Maier‚ Federico Nardi‚ Helge E.S. Pettersen‚ Alberto Ramos‚ Fedor Ratnikov‚ Dieter Röhrich‚ Roberto Ruiz de Austri‚ Pablo Martínez Ruiz del Árbol‚ Oleg Savchenko‚ Nathan Simpson‚ Giles C. Strong‚ Angela Taliercio‚ Mia Tosi‚ Andrey Ustyuzhanin and Haitham Zaraket
In Reviews in Physics. Pages 100085. 2023.
Details about Toward the End−to−End Optimization of Particle Physics Instruments with Differentiable Programming | BibTeX data for Toward the End−to−End Optimization of Particle Physics Instruments with Differentiable Programming | DOI (10.1016/j.revip.2023.100085) | Link to Toward the End−to−End Optimization of Particle Physics Instruments with Differentiable Programming
-
Onboard cloud detection and atmospheric correction with deep learning emulators
Gonzalo Mateo−Garcìa‚ Cesar Aybar‚ Vít Růžička‚ Giacomo Acciarini‚ Atılım Güneş Baydin‚ Gabriele Meoni‚ Nicolas Longépe‚ James Parr and Luis Gómez−Chova
In International Geoscience and Remote Sensing Symposium‚ July 16 – 21‚ 2023‚ Pasadena‚ CA. 2023.
Details about Onboard cloud detection and atmospheric correction with deep learning emulators | BibTeX data for Onboard cloud detection and atmospheric correction with deep learning emulators | Link to Onboard cloud detection and atmospheric correction with deep learning emulators