AI that automatically detects methane plumes from orbit could be a powerful tool in combating climate change
Posted: 17th November 2023
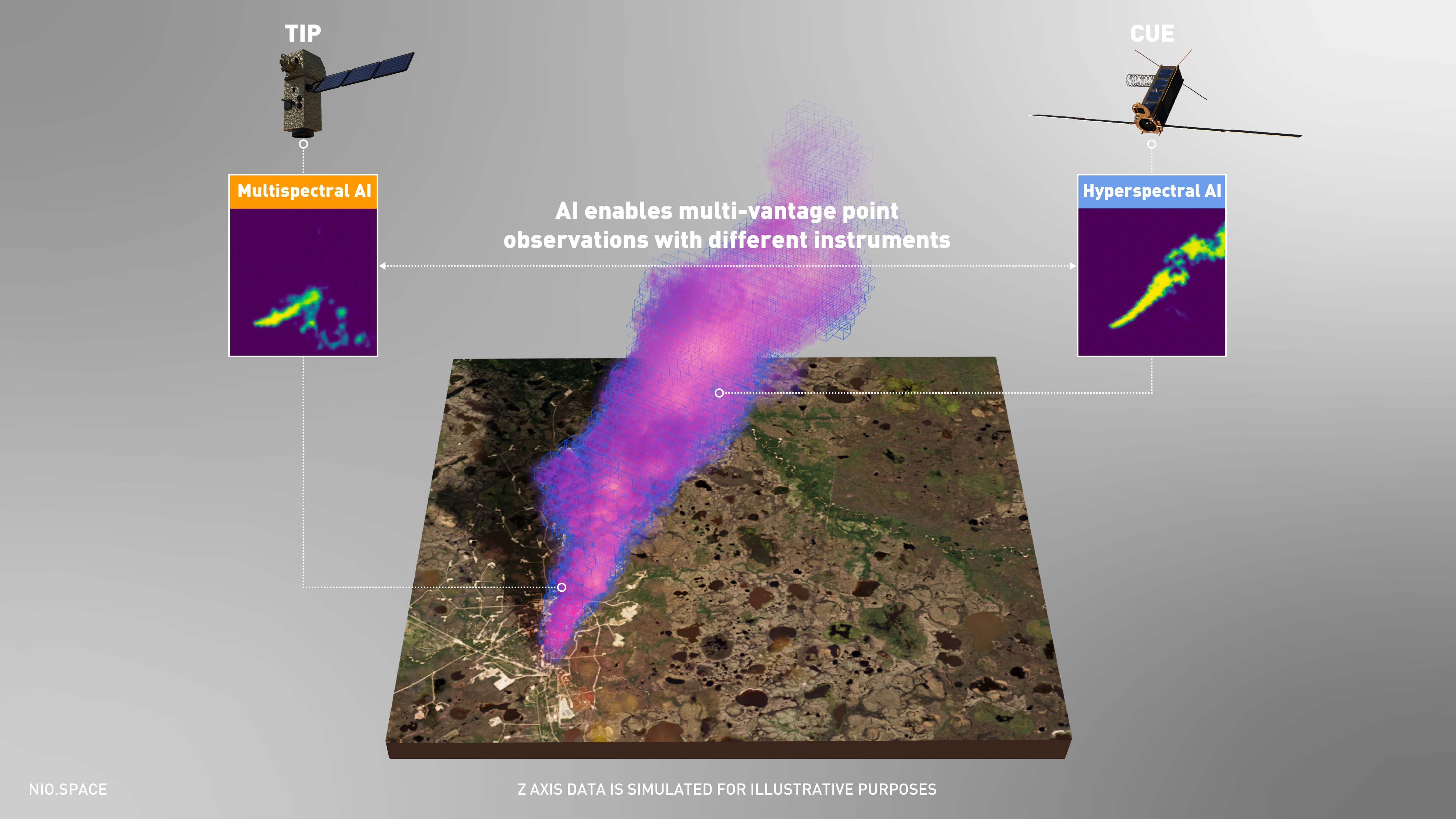
For the first time, University of Oxford researchers, in partnership with Trillium Technologies’ NIO.space have developed a machine learning tool to automatically detect methane plumes on Earth from orbit using machine learning with hyperspectral data from the NASA’s new EMIT sensor. The AI can identify excessive ‘super emitters’ from hyperspectral satellites and could enable more effective action to reduce gas (GHG) emissions and achieve net-zero targets by allowing satellites to work together. The findings have been published today in the journal Nature Scientific Reports.
Although net zero targets focus on carbon dioxide emissions, action on combating methane emissions remains a much faster path to slowing rising temperatures. Methane is 80 times as effective in trapping heat as CO 2 , however it has a much shorter atmospheric lifetime (around 7 to 12 years compared to centuries). Acting quickly to reduce methane emissions from anthropogenic sources would have an immediate impact on slowing global heating and improving air quality.
It has been estimated that readily achievable methane emission reductions could deliver nearly 0.3° C of avoided warming over the next two decades.
Until now, however, there have been only very few methods to readily map methane plumes from aerial imagery and the processing step is time-consuming. This is because methane gas is transparent to both the human eye and the spectral ranges used in most satellite sensors. Even when satellite sensors operate in the correct spectral range to detect methane, the data is often obscured by noise, requiring laborious manual approaches to effectively identify the plumes.
The new machine-learning tool overcomes these issues by detecting methane plumes in data from hyperspectral satellites. These detect narrower bands than more common multispectral satellites, making it easier to tune to the specific signature of methane and filter out noise. However, the amount of data they produce is much larger, making it challenging to process without AI.
The research

The researchers trained the model using 167,825 hyperspectral tiles (each representing an area of 1.64 km2) captured by NASA’s aerial sensor AVIRIS over the Four Corners area of the USA. The algorithm was then applied to data from other hyperspectral sensors in orbit, such as data collected from NASA's new hyperspectral sensor EMIT (Earth Surface Mineral Dust Source Investigation mission) which is attached to the International Space Station and provides near global coverage of the Earth.
Overall, the model has an accuracy of over 81% for detecting large methane plumes*, and was 21.5% more accurate than the previous most accurate approach. The method also had a significantly improved false positive detection rate for tile classification, lowering it by about 41.83% in comparison with the previous most accurate approach.
Lead researcher DPhil student Vít Růžička (Department of Computer Science, University of Oxford) said: ‘What makes this research particularly exciting and relevant is the fact that many more hyperspectral satellites are due to be deployed in the coming years, including from the European Space Agency, NASA, and the private sector. In combination, these new sensors will provide global hyperspectral coverage, enabling worldwide automated detection of methane plumes.'
To promote further research in methane detection, the researchers have open sourced both the annotated dataset and the code used for the model on the project page at GitHub . They are now exploring whether the model could operate directly on-board the satellite itself, allowing other satellites to conduct follow-up observations as part of the NIO.space initiative. This is sometimes referred to as ‘tip and cue’.
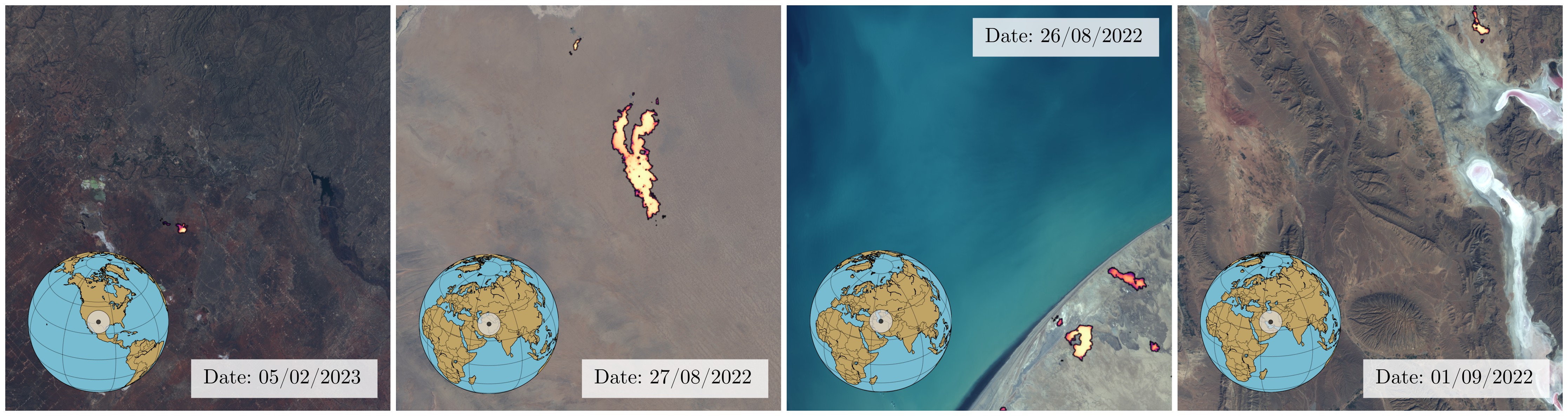
‘Such on-board processing could mean that initially only priority alerts would need to be sent back to Earth, for instance a text alert signal with the coordinates of an identified methane source’ Department of Computer Science DPhil student Vít Růžička
He said: ‘Additionally, this would allow for a swarm of satellites to collaborate autonomously: an initial weak detection could serve as a tip-off signal for the other satellites in the constellation to focus their imagers on the location of interest.’
Professor Andrew Markham who supervised this research said: ‘This new technique will allow rapid and automatic detection of methane emissions, including much smaller plumes, rather than just the `super-emitters’.’
‘In the face of climate change, these kinds of techniques allow independent, global validation about the production and leakage of greenhouse gases. This approach could easily be extended to other important pollutants, and building on earlier work, our ambition is to run these approaches on-board the satellites themselves, making instant detection a reality.’ Department of Computer Science Professor Andrew Markham
The project was funded by the European Space Agency (ESA) Φ-lab via the ‘Cognitive Cloud Computing in Space’ (3CS) campaign and conducted as part of the Trillium Technologies initiative Networked Intelligence in Space ( NIO.space ).